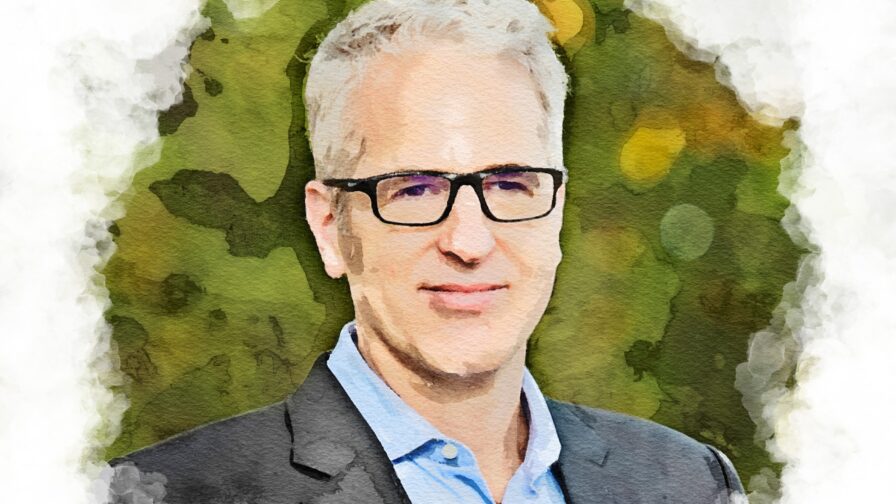
Probabilistic Doing: Eric Siegel on Deploying Machine Learning Projects
By Molly Mitchell
At the culmination of his one-year appointment, Eric Siegel, Darden’s inaugural Bodily Bicentennial Professor in Analytics, gave a final presentation during Reunion Weekend at The Forum Hotel titled “Machine Learning Is Notoriously Difficult to Deploy — But Here’s How.”
Siegel is a leading consultant and former Columbia University professor who focuses on machine learning. He is the founder of the Predictive Analytics World and Deep Learning World conference series, executive editor of “The Machine Learning Times” and author of the bestselling book “Predictive Analytics: The Power to Predict Who Will Click, Buy, Lie, or Die.”
“Business needs prediction,” Siegel said. “Prediction requires machine learning. And machine learning depends on data. So, putting that in reverse, you have data, you give it to machine learning, and it generates models that predict … and those predictions improve all the main things that we do. They render more effective all the large-scale operations of organizations across sectors, which is why we also sometimes refer to machine learning as predictive analytics.”
Siegel explained that, although machine learning is widely acknowledged as a powerful tool for business, these projects often fail at the implementation stage. He attributed this pattern to a lack of standardized business practice for predictive analysis projects and suggested that business professionals with at least semi-technical understanding of machine learning will be more successful. “In other words,” he said professionals must be able to answer, “what does it mean to systematically act on probabilities many times to improve operations?” In his lecture, he provided a six-step practice for running machine learning projects.
But before any step-by-step practice will work, organizations need more people who understand and embrace probabilistic thinking — and probabilistic doing — than “just some data scientists in the corner, doing an analysis.”
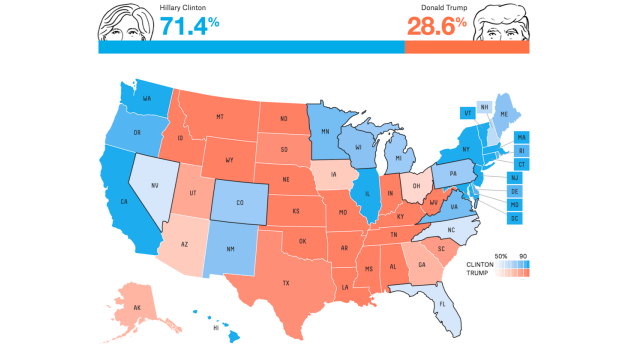
Graph via fivethirtyeight.com. Silver’s probabilities indicated uncertainty, but were widely misinterpreted.
But that’s easier said than done. He used the backlash faced by predictive analyst Nate Silver after the 2016 U.S. presidential election as an example of a common misunderstanding of probability. When Silver gave Donald Trump a 28.6% chance of winning and Hillary Clinton a 71.4% chance, many criticized his model when Trump won. But, Siegel writes in his presentation, “Something that happens 30% of the time is pretty common and normal. And that’s what a probability is. It means that, in a situation just like this, it will happen 30 out of 100 times, that is, 3 out of 10 times. Those aren’t long odds.” Silver’s model, he writes, “expressed, first and foremost, uncertainty. But unfortunately for him, his model’s probability was widely misinterpreted as a definitive prediction.” This confusion underscores the importance of clarifying what a model predicts, its degree of success, and how to act on its predictions.
When business professionals and leaders can metabolize this semi-technical understanding, they can more successfully use Siegel’s six step approach to implementing machine learning projects and understand the process as a change-management process as well. Siegel outlined the six steps as follows: establish the deployment goal, establish the prediction goal, determine the metrics, prepare the data, train the model, and finally, deploy the model. While most of the popular discourse centers around the training step, he emphasized the importance of truly understanding each step, especially what prediction means in this context.
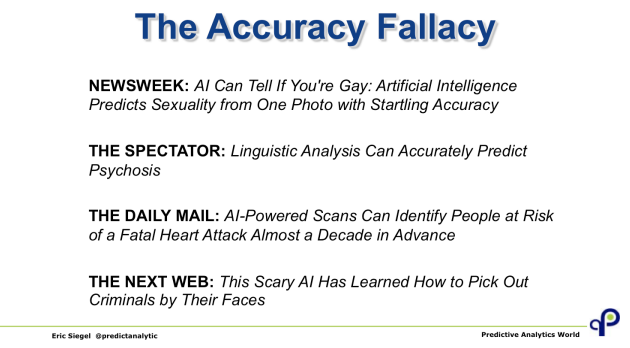
Examples of misleading headlines about machine learning and AI predictive capabilities.
“Unfortunately, the word accuracy always comes up,” he said. “Many human behaviors are not predictable with high confidence. We don’t have magic crystal balls, and we can’t expect the computer to either.” The goal is not necessarily a very high level of accuracy for each individual prediction, but rather a percentage of correct predictions that performs better than guessing. Once you have that, businesses can act on that information that cumulatively results in better performance and therefore a better bottom line.
Ultimately, Siegel urged the audience to reconsider their approach to machine learning projects. Rather than seeing these initiatives as purely technical, they should be viewed as business projects with a technical component. This reframing can help organizations better align technological capabilities with business goals, ensuring more successful deployments and maximal value.
The University of Virginia Darden School of Business prepares responsible global leaders through unparalleled transformational learning experiences. Darden’s graduate degree programs (MBA, MSBA and Ph.D.) and Executive Education & Lifelong Learning programs offered by the Darden School Foundation set the stage for a lifetime of career advancement and impact. Darden’s top-ranked faculty, renowned for teaching excellence, inspires and shapes modern business leadership worldwide through research, thought leadership and business publishing. Darden has Grounds in Charlottesville, Virginia, and the Washington, D.C., area and a global community that includes 18,000 alumni in 90 countries. Darden was established in 1955 at the University of Virginia, a top public university founded by Thomas Jefferson in 1819 in Charlottesville, Virginia.
Press Contact
Molly Mitchell
Associate Director of Content Marketing and Social Media
Darden School of Business
University of Virginia
MitchellM@darden.virginia.edu